Agenda
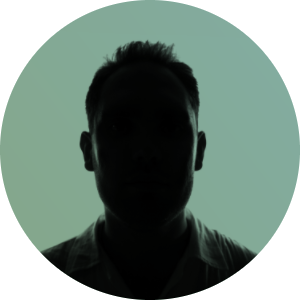
Michael Ruckman
President & CEO
Senteo
• The Difficulties & Imperatives of Leadership in the Digital Age • Evolution of Business Models & Management Models - Product-Centric > Customer-Centric > Relationship-Centric • Changes in Behavior & Difficulties with Engagement • Using Data, Social Context, and Game Theory to Influence Behavior • How Leaders Must Evolve Together with Business Models
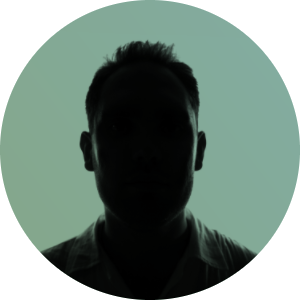
Konstantine Khoperia
Head of BI
TBC Bank
Based on the real example of TBC Bank, we will see how analytical transformation is done in large organizations. How to set objectives, create the proper organizational structure and ensure the systemic development of the transformation program to get the fastest and most sustainable financial results. Following the examples of specific projects, on this highly compelling and challenging way, we will learn how to use data to enhance existing processes and products, generate new ideas, and increase sales.
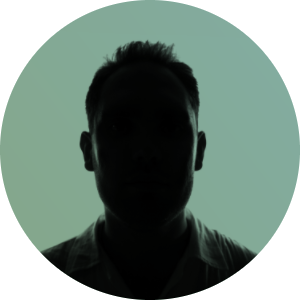
Kevin Sigliano
Professor
IE Business School
Today, customer journeys are vastly collaborative and data-driven. Companies must develop digital excellence strategies if they wish to grow and increase financial return. Therefore, business leaders must understand the drivers, design innovative digital transformation and big data strategies that are impacting financial ROI and business traction. It is crucial to disrupt value propositions and consumer-centric dynamics if you wish to dramatically improve the way your company engages customers and maximize financial results.
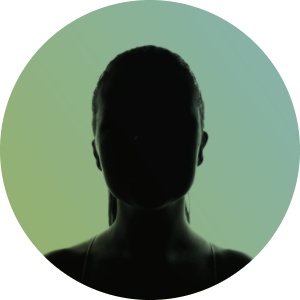
Nutsa Abazadze
Senior Data Scientist
TBC Bank
This workshop is designed to give you a small taster into the large field that is known as machine learning. Using interactive R Markdown notebooks, we will cover some of the most popular supervised learning algorithms for regression and classification tasks. In the first part of the workshop we will focus on linear and penalized regression models. Learn how to solve the problems of overfitting and feature selection using lasso regression, ridge regression and elastic net by applying a very useful package ‘glmnet’. For better interpretability we will visualize our models and do some interactive visualizations using the package ‘coefplot’.
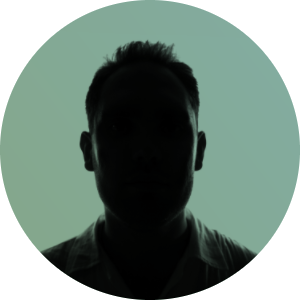
Serhat Keçici
Cognitive and Analytics Practice Lead
IBM
Neural Networks are different than other machine learning models, they are algorithmically more difficult to handle, yet more capable to address complex real-life problems. Neural networks are very important today and will be even more important tomorrow. As data scientists and data science leaders we need to understand how neural networks works in order to benefit from this already emerged area of machine learning. In this session we will address this by covering the neural networks theory by keeping a nice balance between theory and the rationale behind. It will be a good rapid start for the ones who are new to neural networks, and a supplementary discussion for the ones already familiar with Neural Networks. Here are some keywords from the session: NN Layers and units, activation function, backpropagation, gradient descent, drop-out, NN Types (CNN, RNN etc.).
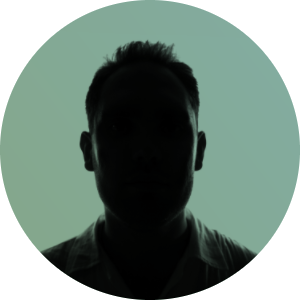
Valeriy Babushkin
Director of Data Science
X5 Retail Group
Machine learning allows us to interpret data structures and fit that data into models to identify patterns and make predictions. Python makes this easier with its huge set of libraries that can be easily used for machine learning. It is essential to keep upgrading your machine learning skills as there are immense advancements taking place every day. In this course, you will get hands-on experience of solving real problems by implementing cutting-edge techniques to significantly boost your Python Machine Learning skills and, as a consequence, achieve optimized results in almost any project you are working on. Each technique we cover is itself enough to improve your results. However; combining them together is where the real magic is. Throughout the course, you will work on real datasets to increase your expertise and keep adding new tools to your machine learning toolbox. By the end of this course, you will know various tips, tricks, and techniques to upgrade your machine learning algorithms to reduce common problems, all the while building efficient machine learning models.
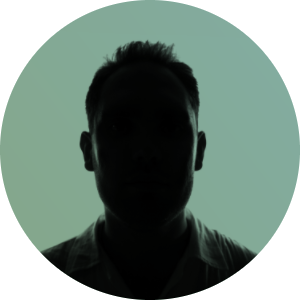
Carlo Giovine
Associate Partner
QuantumBlack
Carlo will explore advances in analytics over recent years, and highlight real examples of where this is being applied at scale. He will share some of the learnings gathered in QuantumBlack from over a decade of cross-industry experience in applying AI to business problems..
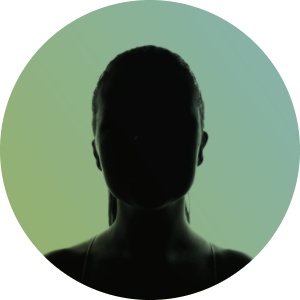
Nino Masurashvili
Deputy CEO, Chief Risk Officer
TBC Bank
TBC Bank is the largest bank in Georgia with more than two million clients in the country. The Bank's aspiration is to grow to 10 million users in three countries by 2022. The leadership of the bank bets this expansion on two main instruments: digital - to scale quickly, and analytics - to know customers better. TBC Bank's analytical transformation is the integral part of this larger push. With 6 analytical transformation projects running in parallel at any given time, it has become an essential instrument for transforming bank's core businesses and making them more customer-centric and profitable. Join us as we explore how the transformation was built and implemented, and what successes and learning have been accumulated from it.
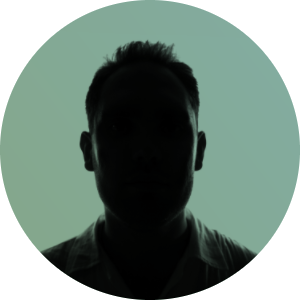
Rudradeb Mitra
Founder
Omdena
87% of AI solutions don't go into production, and this puts a big question mark on the future of AI. In this talk, the speaker will share his experiences in building successful AI solutions. He will argue why collaboration is the key to building ethical, sustainable, and trustworthy AI solutions, that will lead to successful end-user adoption. He will then share his 3-step processes that companies can use to create collaborative environment and successfully build AI solutions.
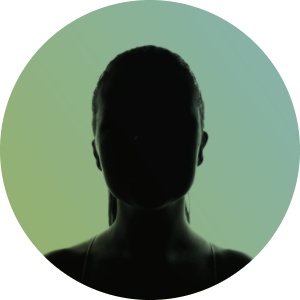
Mariam Melikadze
Director, Data Insights Group
New York Times
The "cookiepocolypse" is coming. With 3rd party cookies going away and additional scrutiny on privacy, advertisers and publishers are trying to find alternative ways to target ads effectively. Join us for this talk to hear how the New York Times is planning for the future by using machine learning and onsite reader behavior data to build privacy-friendly audience segments. Mariam will discuss how the team progressed from an initial idea to building production-grade products and the things they learned along the way.
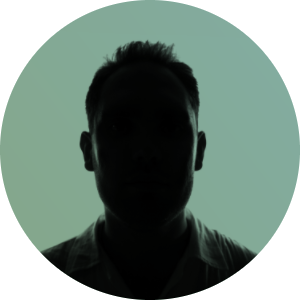
Levan Borchkhadze
Senior Data Scientist
TBC Bank
TBC Bank is in transition from product centric to client centric company. As such, one of the most obvious applications of analytics is developing personalized and automated next best product recommendation for clients. Successful applications of recommender engines in retail banking are still rare, but potential benefits are enormous. After considering various collaborative filtering approaches we decided to implement ALS user-item matrix factorization method and demographic model. There are no explicit ratings available that can be exploited by a recommender system for retail banking which makes modeling more complicated. Main challenge was to handle implicit data and un-homogenous nature of our industry features in contrast to entertainment businesses. After multiple online test iterations we obtained surprisingly good results which was well comparable with offline tests. As a result, customer tailored offers boosted sales and profitability, and increased customer satisfaction at the same time.
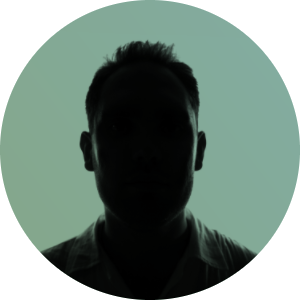
George Chkadua
Data Scientist
TBC Bank
TBC Bank is in transition from product centric to client centric company. As such, one of the most obvious applications of analytics is developing personalized and automated next best product recommendation for clients. Successful applications of recommender engines in retail banking are still rare, but potential benefits are enormous. After considering various collaborative filtering approaches we decided to implement ALS user-item matrix factorization method and demographic model. There are no explicit ratings available that can be exploited by a recommender system for retail banking which makes modeling more complicated. Main challenge was to handle implicit data and un-homogenous nature of our industry features in contrast to entertainment businesses. After multiple online test iterations we obtained surprisingly good results which was well comparable with offline tests. As a result, customer tailored offers boosted sales and profitability, and increased customer satisfaction at the same time.
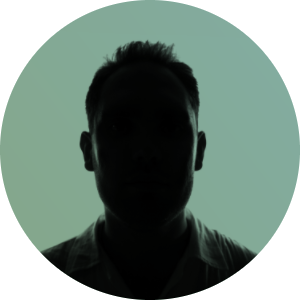
Aleksander Djurka
Chief Information Officer
Vestiaire Collective
Every Data manager faced with the challenge of setting up a platform from scratch has to face multiple technical decisions, in order to build the best possible architecture for their own companies. In order to accomplish that, we need to balance cost, time and effectiveness. The capabilities of tools offered on the open market are increasing every day, with the large cloud providers competing who can provide the most complete, efficient and easy-to-implement (and difficult to move out of) data solutions. We will explore the cloud building blocks that we can use from the currently available tech, and together discover the architecture to enable Data Warehousing, deployments of Data Science algorithms and real-time computing pipelines.
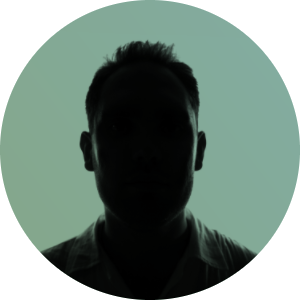
Mattia Boni Sforza
Principal Data Scientist
QuantumBlack
Experts and practitioners in various domains are commonly interested in discovering causal relationships to answer questions like “What drives economical prosperity?”, “What fraction of patients can a given drug save?”, “How much would a power failure cost to a given manufacturing plant?”. The ability to identify truly causal relationships is fundamental to developing impactful interventions in medicine, policy, business, and other domains. Often, in the absence of randomised control trials, there is a need for causal inference purely from observational data. However, in this case the commonly known fact that “correlation does not imply causation” comes to life. Therefore, it is crucial to distinguish between events that cause specific outcomes and those that merely correlate. This talk will focus on using CausalNex, recently open-sourced Python package for Causal Inference from QuantumBlack. This library is the output of learning and collaboration between our R&D teams, client engagements, and QuantumBlack Labs. CausalNex focusses on applying Bayesian Networks, and driving a collaborative approach between Data Scientists and Domain Experts.
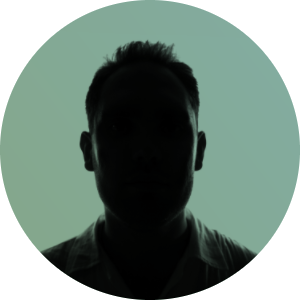
Serhat Keçici
Cognitive and Analytics Practice Lead
IBM
Deep learning is changing how we do analytics, it is different than other M/L algorithms and it is capable to handle much more complex problems. In this session we will address how deep learning is different, how it is used, why we call it as a potential disruption in analytics and how we should respond to that as data scientists and data science leaders..
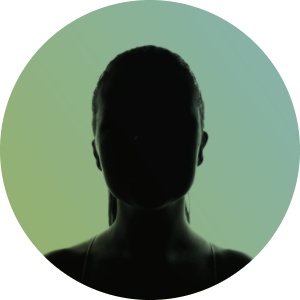
Violeta Misheva
Data Scientist
ABN Amro Bank N.V
Explainability of ML and AI models has been rising in popularity with the growth of ML and AI applications. There are now many approaches to explain the decision and behavior of a ML model. In this talk, we we define explainability and why it is important before we review some of the more popular and promising methods, applied to a use case. The talk will end with sharing some best practices from applying explainability frameworks in the finance industry.
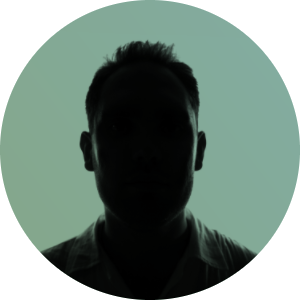
Kevin Sigliano
Professor
IE Business School
Discover how to transform your company's marketing strategy with effective customer-centric digital tactics maximizing business performance results. All sectors must adopt social media, paid and e-commerce innovative performance-driven tactics based on fast-changing consumer habits and preferences. Discover the most effective Digital Marketing framework and how to maximize your Digital ROMI.
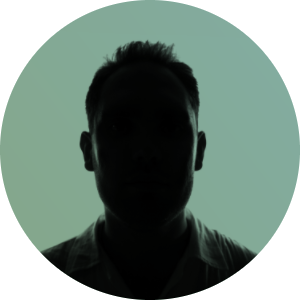
Abhijit Akerkar
Head of AI Business Integration
Lloyds Bank
Over $35bn have been invested into AI by companies around the world in the last year alone. But two in every three of those companies are yet to generate any tangible value. And of those companies that have turned the corner, almost half are still at the sub-scale level. The good news is that value is not elusive. Companies such as Google and Amazon generate over 50% of their profits using AI. How can your company crack the code to conquer those AI riches?
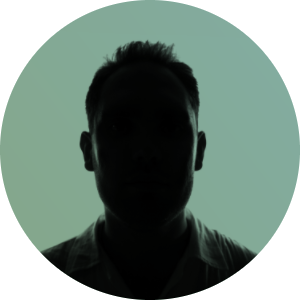
Andreas Galatoulas
Chief Technology and Innovation Officer
Barking & Dagenham College
The aim of this talk is to help you understand and explain advance machine learning models to Executives in a high regulated environment. We answer the key question: Can we fully explain a predictive model and what are the sacrifices we will have to make.
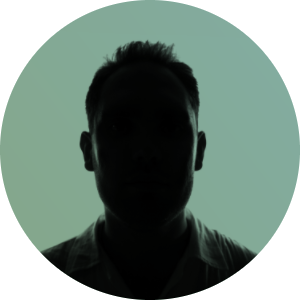
Giorgi Mirzikashvili
Co-Founder
OptioAI
An innovative approach to financial data classification is one of the most important ways to precisely understand customers’ spending behavior and their future financial performance. During the presentation, we will explore technical and business aspects of leveraging the power of Natural Language Understanding in the process of data classification and also find what impact can have a network effect in the fintech products
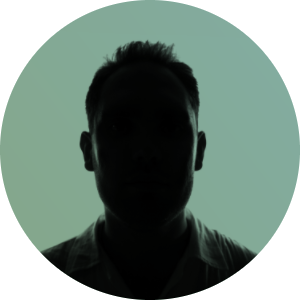
Irakli Menabde
Chief Analytics Officer
Cashflows
Irakli will discuss analytics frameworks and performance metrics used to gain visibility and enhance a company’s sustainable competitive advantage to create business value in todays rapidly growing fintech payment space. That includes both data driven, operational effectiveness and strategic positioning by gaining insight to identify new approaches to innovation and differentiation. The presentation will focus on two actual case studies of Onboarding and Risk Management at Cashflows. CashFlows is majority owned by the Private Equity Fund Pollen Street Capital and is a fast-growing and innovative provider of merchant payment solutions in the fintech space based in London. Focused on maximizing payment success, CashFlows has an aggressive growth trajectory and currently processes payments for more than 1,500 customers and powers over 34,000 ATMs across Europe. Irakli currently serves as the Chief Analytics Officer since 2017 and is a member of the Board.
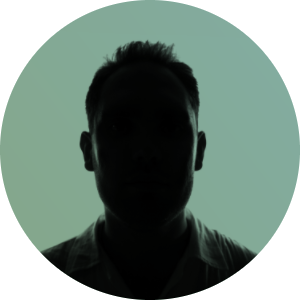
Vincenzo Lagani
Co-Founder
Gnosis Data Analysis
Identifying the most important variables is often the actual goal of predictive analysis. For example, insurance companies may desire to know which factors influence customer attrition, so that to proactively decrease the churn rate. In marketing, strategists may be interested in knowing whether they should intensify social media presence rather than email messaging in order to increase a campaign effectiveness. Unfortunately, intervening on variables that are important for prediction does not always results in the desired change, because those variables may be merely associated with the outcome, and not a cause of it. Experimental procedures like A/B testing can help in discerning causes from associations, but in some sectors they can be lengthy and costly. Causal Analysis is a relatively novel field that attempts to identify causal relationships from historical data without resorting to expensive experiments. In this talk we will discuss about the state-of-the-art of causal analysis, with particular regards to the applicability of its methods in real-world settings.
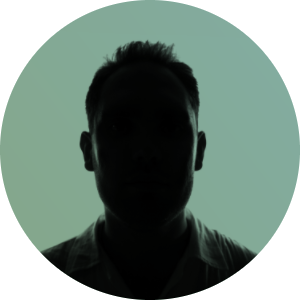
Dmitry Soshnikov
Sr. Cloud Developer Advocate
Microsoft
Modern neural language models can handle a lot of different tasks, from simple text classification to complex ones, such as summarization and question answering. Open Domain Question Answering (ODQA) refers to the models that can answer open questions on a large text corpus, such as Wikipedia. In this talk, I show you how to set up text processing pipeline for ODQA using DeepPavlov NLP framework and Azure Machine Learning, and how to train the model to answer questions from CORD dataset of scientific papers on COVID infection.
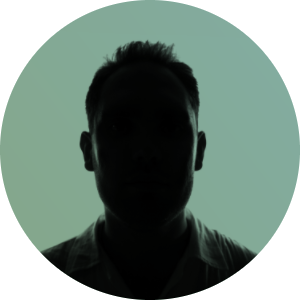
George Popov
AI Software Developer
Neiron
Modern organizations are focused on the quality of their products and services more than on anything else. It is easily understandable that customers are more likely to buy top quality goods and services. Quality control is crucial when it comes to market competition. Thus, Neiron is helping a Georgian snacks brand FRIXX to assure the quality of its products using Computer Vision, Deep Learning, and Data Analysis. Combinations of different Deep Convolutional Neural Networks are used to classify bad products from good. Other state-of-the-art models do instance segmentation on a real-time video stream from the factory. Neiron believes that AI is the right way to improve quality control in any existing industry.